Mathematical Statistics and Data Science
The research group in Mathematical Statistics and Data Science studies advanced methods and models for analysing and representing data. We employ probability theory and stochastic processes to rigorously model uncertainty and randomness, and abstract and linear algebra to understand the structure of statistical models and the relationships between their parameters.
News and events
Join
stochastics@list.aalto.fi to stay updated on probability and statistics in Aalto University.
Join
stochastics-finland@list.aalto.fi for announcements on probability and statistics in Finland.
Members
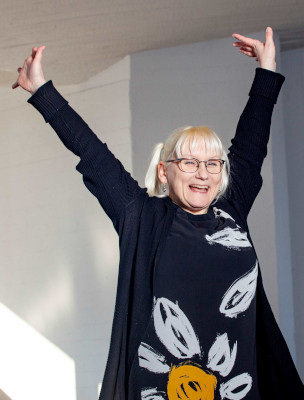 |
Pauliina Ilmonen Associate Professor Multivariate extreme values, functional data analysis, cancer epidemiology |
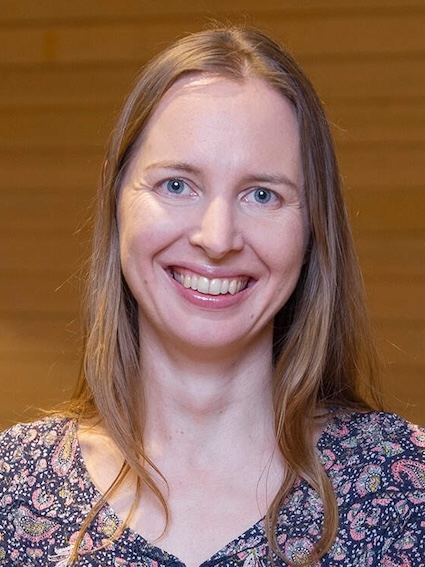 |
Kaie Kubjas Associate Professor Algebraic statistics |
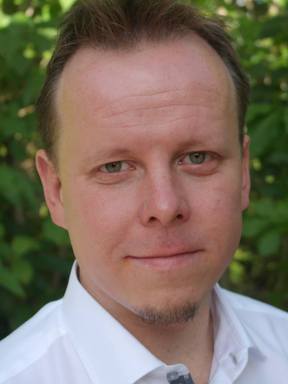 |
Lasse Leskelä Associate Professor Mathematical statistics, network analysis, probability theory |
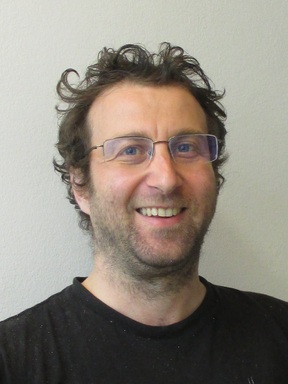 |
Vanni Noferini Associate Professor Network analysis, random matrix theory |
Publications
Individual publication records and links to full articles when available can be found on the
Aalto research page, where you can also find an overview of
research output for the Mathematical Statistics and Data Science area.
Selected publications
-
- F Arrigo, DJ Higham, V Noferini, R Wood. Weighted enumeration of nonbacktracking walks on weighted graphs. SIAM Journal on Matrix Analysis and Applications 2024.
- M Bloznelis, L Leskelä. Clustering and percolation on superpositions of Bernoulli random graphs. Random Structures & Algorithms 2023.
- M Hinz, JM Tölle and L Viitasaari. Variability of paths and differential equations with BV-coefficients. Annales de l’Institut Henri Poincaré - Probabilités et Statistiques 2023.
- A Belyaeva, K Kubjas, LJ Sun, C Uhler. Identifying 3D genome organization in diploid organisms via Euclidean distance geometry. SIAM Journal on Mathematics of Data Science 2022.
- J Alho, E Arjas, J Karvanen, L Leskelä, E Läärä ja P Pere. Tilastotieteen sanasto. Suomen Tilastoseura 2023.
Teaching
We teach courses in probability and statistics at all levels. Some of the offered courses are eligible as a basis for an
SHV degree in insurance mathematics. Doctoral education in probability and statistics is coordinated by the
Finnish Doctoral Education Network in Stochastics and Statistics (FDNSS).
Seminars
Upcoming seminars
Projects and networks
Page content by: webmaster-math [at] list [dot] aalto [dot] fi